Machine learning can teach computers to learn information and improve. Here’s what it is and how to invest in the sector.
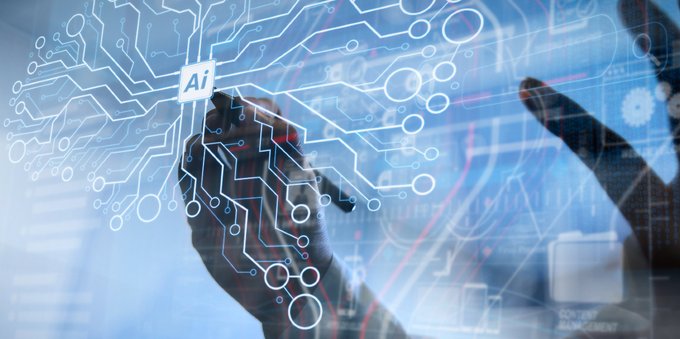
Although there is a lot of talk about artificial intelligence today, the concept of machine learning is still completely unknown to many people. It is important to know this because many digital and AI-related aspects of our lives are related to machine learning.
In recent years, it is no coincidence that so-called automatic learning (Machine Learning, ML) has transformed industrial sectors, from healthcare to finance, through marketing and automation. This technology is part of the broader sphere of artificial intelligence and has become a key factor in technological innovation. But what exactly is machine learning and why is it becoming so relevant? Here’s what to know, also - or perhaps especially - for those who intend to invest in the sector.
What is machine learning? Meaning and Definition
Machine learning allows computers to learn autonomously from data and improve their performance without being explicitly programmed. This concept is based on the idea that systems can identify patterns in data, analyze information, and make decisions or predictions autonomously.
The impact of this technology is evident in search engines, recommendation systems, voice recognition, and even self-driving cars.
Machine learning is synonymous, in Italian, with automatic learning and is a very important branch of artificial intelligence. In other words, it is a method that allows machines to gain experience and improve their performance by analyzing their given information.
In machine learning, algorithms - which represent the main engine - are “trained” to classify objects, identify patterns, make predictions, and make advantageous decisions.
How Machine Learning Works
There are four types of learning in machine learning: supervised, unsupervised, semi-supervised, and reinforcement.
1. Supervised Learning
This is the first of the four machine learning models. With supervised learning, the machine receives a set of data already labeled as input and output and begins to analyze them through algorithms to derive the relationships between them. Once the training is finished, if you give the machine an input similar to those used previously, it should be able to provide a consistent output.
An example of supervised learning is with the recognition and classification of images. For example, imagine having to classify animals that stand on two or four legs based on images. The training data should be correctly labeled to identify whether an animal stands on two or four legs. After that, the machine will be able to proceed autonomously.
2. Unsupervised learning
Unsupervised learning provides the machine with only a set of data, some unlabeled and some labeled as input.
At this point, it is up to the machine to provide coherent outputs and to derive the relationships present through the algorithms. This learning method is particularly useful when you have a large data set and you want to try to discover new relationships between the data that you are not aware of.
We deal with unsupervised learning whenever we interface with recommendation systems, such as those on e-commerce sites. In particular, based on our research, the systems can also suggest other products that have been purchased by people who have searched for something similar.
3. Semi-supervised learning
This machine learning model is halfway between supervised and unsupervised.
In this model, the semi-supervised learning algorithm instructs the machine to analyze the labeled data to detect features that could also be present in the unlabeled data.
As for semi-supervised learning, one of the most popular application examples has to do with fraud detection in financial transactions. In fact, thanks to training carried out on a series of labeled data, the machine is able to obtain patterns with which to track down any possible fraud in the sector. If this happens, the machine can trigger an alarm that puts people in a position to do the necessary investigations.
This type of learning also presents risks, in fact, if the data with which the machine has been trained are wrong, it risks repeating the same errors even in unlabeled data.
4. Reinforcement learning
Compared to other machine learning models, this is different, since it is not based on associating labeled and unlabeled data. Reinforcement learning aims to create autonomous agents capable of choosing which actions to perform to achieve certain objectives through interaction with the environment in which they are immersed.
For each action performed, the machine receives a feedback, positive or negative. If this is positive, the machine will memorize this behavior, otherwise it will look for a new solution. As training progresses, the machine should become increasingly autonomous, until it becomes independent.
This type of training is used to train cars equipped with advanced driver assistance systems. Through training, these cars can learn the best conditions to perform a maneuver, such as changing lanes or overtaking.
leggi anche
Forget AI. The next megatrend will be robotics
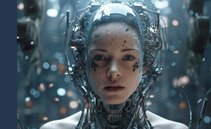
The role of machine learning in AI: the current state
Machine learning plays a crucial role in modern AI, as it forms the basis for the development of algorithms that can automatically improve over time.
AI is a very broad field that includes machine learning, deep learning, computer vision, natural language processing (NLP) and more. However, machine learning is currently one of the most effective techniques for making AI operational and scalable.
Role of machine learning
Today, machine learning is the basis for many of the AI applications we use every day. Some examples include:
- intelligent voice assistants like Siri, Alexa and Google Assistant, which use machine learning algorithms to recognize and understand natural language;
- recommendation engines like those of Netflix, Amazon or Spotify, which analyze large amounts of data about users to suggest personalized products or content;
- fraud detection systems: In banks and financial institutions, machine learning models analyze transactions in real-time to detect fraudulent patterns.
Machine learning and Big Data
One of the most significant developments is the intersection of machine learning and Big Data. The increasing availability of huge volumes of data (Big Data) has allowed machine learning models to become more accurate and predictive. This data includes everything from social media interactions to sensory data collected by IoT (Internet of Things), which opens the way to new applications in areas such as predictive maintenance, medical diagnosis, and personalized marketing.
Current Applications
Machine learning has already transformed many industries:
- healthcare: Machine learning algorithms are used to analyze medical images, improving the diagnosis of diseases such as cancer;
- automotive: Self-driving cars, such as those developed by Tesla and Waymo, rely heavily on machine-learning models to make real-time decisions;
- finance: In addition to fraud detection, machine learning is used to analyze financial markets, optimize portfolios, and predict price movements.
How to invest in machine learning?
As we have seen, machine learning plays an increasingly important role in today’s technological world. Thanks to it companies can create predictive models or autonomous agents and consequently improve their business.
To invest in this sector it is first of all essential to have a clear understanding of how it works and what laws regulate it.
Once this is done, it is possible to search for companies, including startups, that apply it, focusing on their stock price. Since the machine learning sector is experiencing a moment of great popularity, it is not difficult to find companies that focus on it to create predictive models or cutting-edge autonomous agents.
After the process of researching the companies in which you intend to invest, it is essential to ensure that they are solid and that the solution they offer has an outlet market. Investing in machine learning, in fact, can be extremely profitable, given the exponential growth of the sector. There are different approaches to doing so, depending on the available capital and risk appetite.
1. Invest in publicly traded companies
- Many leading companies in the field of machine learning are publicly traded. Google (Alphabet), Amazon, Microsoft, NVIDIA, and Tesla are just a few of the large companies that are investing heavily in this technology. Investing in shares of these companies can be an effective strategy for those who want to gain exposure to the sector.
2. Investment funds and ETFs
- A more diversified alternative to buying individual shares is to invest in investment funds or ETFs specialized in technology and artificial intelligence. These funds collect portfolios of machine learning-related stocks and can reduce the risk compared to investing in a single company.
3. Startups and venture capital
For more experienced investors, there is the opportunity to invest in innovative startups that are developing machine-learning solutions. This type of investment can lead to high returns, but it also carries a higher risk than investing in established companies.
4. Acquiring skills
Finally, investing in machine learning is not just about investing capital. Acquiring skills in this field, through online courses or graduate programs, can lead to well-paying job opportunities. Machine learning skills are in high demand and can provide career growth opportunities in various industries.
Original article published on Money.it Italy 2024-10-23 12:49:49. Original title: Machine learning, cos’è e come funziona l’apprendimento automatico